As the COVID-19 crisis escalated in mid-March, Minnesota Public Radio News Chief Meteorologist Paul Huttner wrote an article comparing everyone’s efforts to predict who would get sick and die to forecasting a storm with a broken weather forecast model.
Mood Music:
Describing the gaping hole in U.S. testing efforts, he wrote:
It’s like one of our weather satellites is down, and we can’t get a clear picture of what the storm looks like from above. We just can’t see the whole storm.
It was an apt analogy then and remains so. Yet we continue to grind our gears over a busted radar and barometer.
Nearly six weeks later, testing is still a massive failure in this country. We still lack the accurate picture needed to build forecasts and make plans to re-open society.
We see an endless array of charts, maps and other data presentations and thousands of articles across the internet that dissect it all in search of clues on how the virus affects the young vs. middle-aged vs. old. There are death statistics for all 50 U.S. states, for Italy, for Spain, and on and on. All this new data, daily.
And without massive testing and contact tracing, sifting through it all and making conclusions are an exercise in futility.
That doesn’t mean the data we have is useless. Every data point offers a lesson that we can use to make smarter decisions — and we have.
But trying to make the big-picture conclusions using data that doesn’t have a solid foundation beneath it? It’s starting to seem like a waste of time and resources.
Truth is, testing and contact tracing will never be where they need to be. There’s not enough personnel, supplies or logistical agility for the former, and the latter is rife with technical glitches. Not to mention the potential for government misuse.
So we’ll never have the broad, solid foundation to put all the other data into the proper context. We’re never going to know the exact number of people around the world sickened with COVID-19. We’ll never know the true death rate.
Perhaps we should make peace with what we don’t know and start figuring out how we can keep the largest number of people as healthy and safe as possible while re-opening businesses, schools and recreation.
Last weekend I read an interesting Wall Street Journal piece by Avik Roy, president of the Foundation for Research on Equal Opportunity and co-author of the foundation’s “A New Strategy for Bringing People Back to Work During Covid-19.” In the essay, Roy notes that we have specific goals we’re trying to make before we go “back to normal,” things like near-universal testing and an approved vaccine. “But,” he writes:
This conventional wisdom has a critical flaw. We’ve taken for granted that our ingenuity can solve almost any problem. But what if, in this case, it can’t? What if we can’t scale up coronavirus testing as quickly as we need to? What if it takes us six or 12 months, instead of three, to identify an effective treatment for Covid-19? What if those who recover from the disease fail to gain immunity and are therefore susceptible to getting reinfected? And what if it takes us years to develop a vaccine?
Such questions can raise fears in us, but these are truth-based fears. We can see, Roy points out, how unrealistically optimistic our goals are. It’s far more likely that we won’t make all our goals. And if we don’t, what then? “Do we prolong the economic shutdown for six months or longer? Do we impose a series of on-and-off stay-at-home orders that could go on for years?”
Roy doesn’t have the answers for how we move forward, but he does offer a starting point I agree with:
Instead of thinking up creative ways to force people to stay home, we should think hard every day about how to bring more people back to work.
Our current analysis paralysis — fixated around data sets that are limited without knowing the bigger picture of who exactly has had the virus, recovered or died from it — is unsustainable.
There’s a way forward. But it’s going to involve us taking a few leaps of faith along the way and tossing aside the broken forecasting tools.
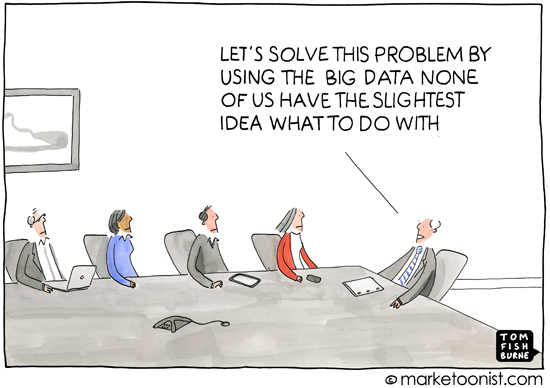
Very good article, Bill. You hit the nail on the head. Whatever the reason, be it technical or political, testing adequate numbers of people is just not happening. You are absolutely correct, how can we make life-and-death decisions without the proper statistics? Do I want to go out of the house and associate with groups of people who could be carriers? Do I already have the antibody so I don’t have to worry as much? Will I ever know the answers to these questions? Unfortunately, your article and every one I have read on this subject does not come to any recommendations that will tell us what to do and when to do it. This is indeed a quagmire.